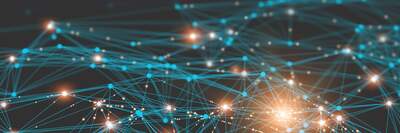
Anwendungsberichte
Automatic isolation of pollen using NIS-Elements General Analysis (GA) and JOBS imaging workflow tools
November 2023
Quantitative 3D Imaging of Living Organs-on-Chips with a High-Speed Point-Scanning Confocal System
Januar 2021