Notes d'application
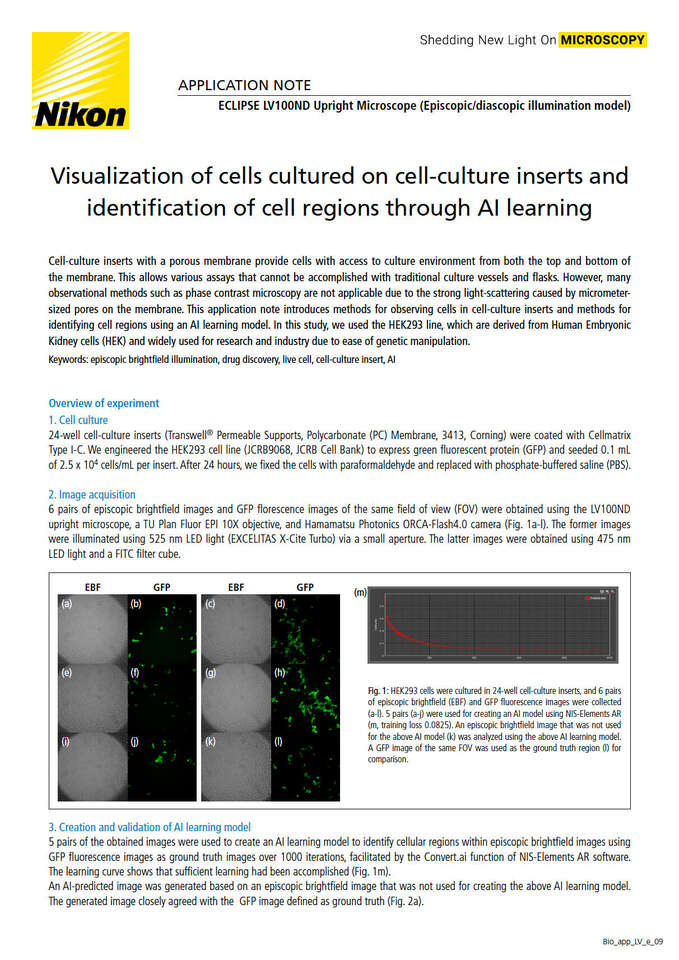
Visualization of cells cultured on cell-culture inserts and identification of cell regions through AI learning
décembre 2023
Cell-culture inserts with a porous membrane provide cells with access to environmental nutrients from both the top and bottom of the membrane. This allows various assays that cannot be accomplished with traditional culture vessels and flasks. However, manyobservational methods such as phase contrast microscopy are not applicable due to the strong light-scattering caused by micrometer-sized pores on the membrane. This application note introduces methods for observing cells in cell-culture inserts and methods foridentifying cell regions using an AI learning model. In this study, we used the HEK293 line, which are derived from HumanEmbryonic Kidney cells (HEK) and widely used for research and industry due to ease of genetic manipulation.
Keywords: episcopic brightfield illumination, drug discovery, live cell, cell-culture insert, AI
Overview of experiment
1. Cell culture
24-well cell-culture inserts (Transwell® Permeable Supports, Polycarbonate (PC) Membrane, 3413, Corning) were coated with Cellmatrix Type I-C. We engineered the HEK293 cell line (JCRB9068, JCRB Cell Bank) to express green fluorescent protein (GFP) and seeded 0.1 mL of 2.5 x 104 cells/mL per insert. After 24 hours, we fixed the cells with paraformaldehyde and replaced with phosphate-buffered saline (PBS).
2. Image acquisition
6 pairs of episcopic brightfield images and GFP florescence images of the same field of view (FOV) were obtained using the LV100ND upright microscope, a TU Plan Fluor EPI 10X objective, and Hamamatsu Photonics ORCA-Flash4.0 camera (Fig. 1a-l). The former images were illuminated using 525 nm LED light (EXCELITAS X-Cite Turbo) via a small aperture. The latter images were obtained using 475 nm LED light and a FITC filter cube.


Fig. 1: HEK293 cells were cultured in 24-well cell-culture inserts, and 6 pairs of episcopic brightfield (EBF) and GFP fluorescence images were collected(a-l). 5 pairs (a-j) were used for creating an AI model using NIS-Elements AR (m, training loss 0.0825). An episcopic brightfield image that was not usedfor the above AI model (k) was analyzed using the above AI learning model. A GFP image of the same FOV was used as the ground truth region (l) for comparison.
3. Creation and validation of AI learning model
5 pairs of the obtained images were used to create an AI learning model to identify cell bodies within episcopic brightfield images using GFP fluorescence images as ground truth images over 1000 iterations, facilitated by the Convert.ai function of NIS-Elements AR software. The learning curve shows that sufficient learning had been accomplished (Fig. 1m).
An AI-predicted image was generated based on an episcopic brightfield image that was not used for creating the above AI learning model. The generated image closely agreed with the GFP image defined as ground truth (Fig. 2a).

Fig. 2: (a) The AI-predicted image and the GFP fluorescence image mostly overlapped. (b) The central parts (white circle, whose diameter is 90% of theimage length and width) were used for image processing and quantitative evaluation. The cell areas of the ground truth region (yellow), the AI-predictedregion (light blue), and the region common to both (pink) were measured.
Image processing was executed on the central portion of the FOV in order to reduce effects of the liquid surface meniscus, and a quantitative evaluation comparing predicted and true cellular regions was conducted (Fig. 2b). The ratio of the ground truth and AI-predicted overlapping region (pink in Fig. 2b) to the ground truth region (yellow in Fig. 2b) was 81%, and the ratio of the overlapping region to the AI-predicted region (light blue in Fig. 2b) was 76%. This indicates that the AI-predicted region is in good agreement with the ground truth region.
Summary
Applying AI to episcopic brightfield images enables evaluation of seeding density and variability of live cells, which had been difficult due to the“visibility problem” caused by the optical characteristics of the culture substrate. This technology can considerably reduce the number of evaluationsamples required for searching culture conditions and can improve experimental efficiency.
Product Information
ECLIPSE LV100ND upright microscope (Episcopic/diascopic illumination model)
The ECLIPSE LV100ND can support brightfield, fluorescence, DIC and polarizing observation using an episcopic illuminator with a high-intensity halogenlamp, in addition to bright-field, phase contrast, DIC and polarizing observation using a diascopic illuminator.
TU Plan Fluor EPI 10X
This CFI60-2 objective, with a high NA (0.3) and long working distance (17.5mm), is optimized for episcopic observation. Sharp, high-contrast imagescan be obtained with excellent chromatic aberration correction.