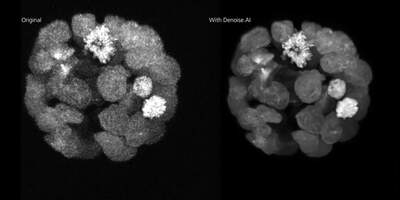
Image courtesy of Laurence Pelletier Lab, LTRI
NIS-Elements AI
Utilizing Deep Learning to remove Poisson shot noise from resonant confocal images
Using a convolutional neural network using an MXNET framework encoded with several thousand examples of resonant confocal data, the input image data is assigned learnable weights, which results in teaching of the network to make correlations and recognize patterns: with the main common pattern being Poisson shot noise, the network was trained to recognize and remove shot noise from resonant A1 confocal data sets. This trained Artificial Intelligence (AI) algorithm can then be used even in real-time for noise removal.
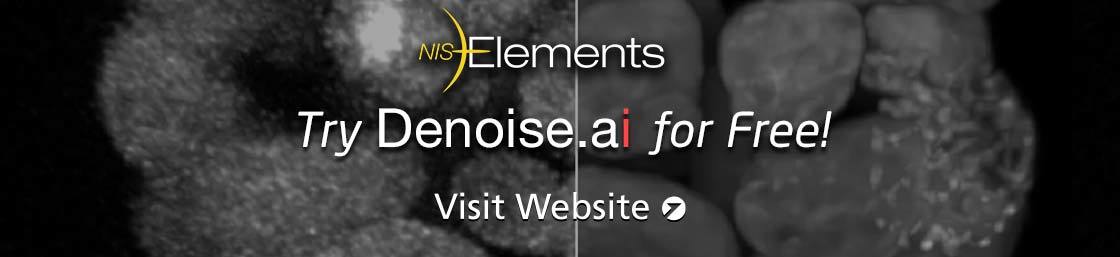
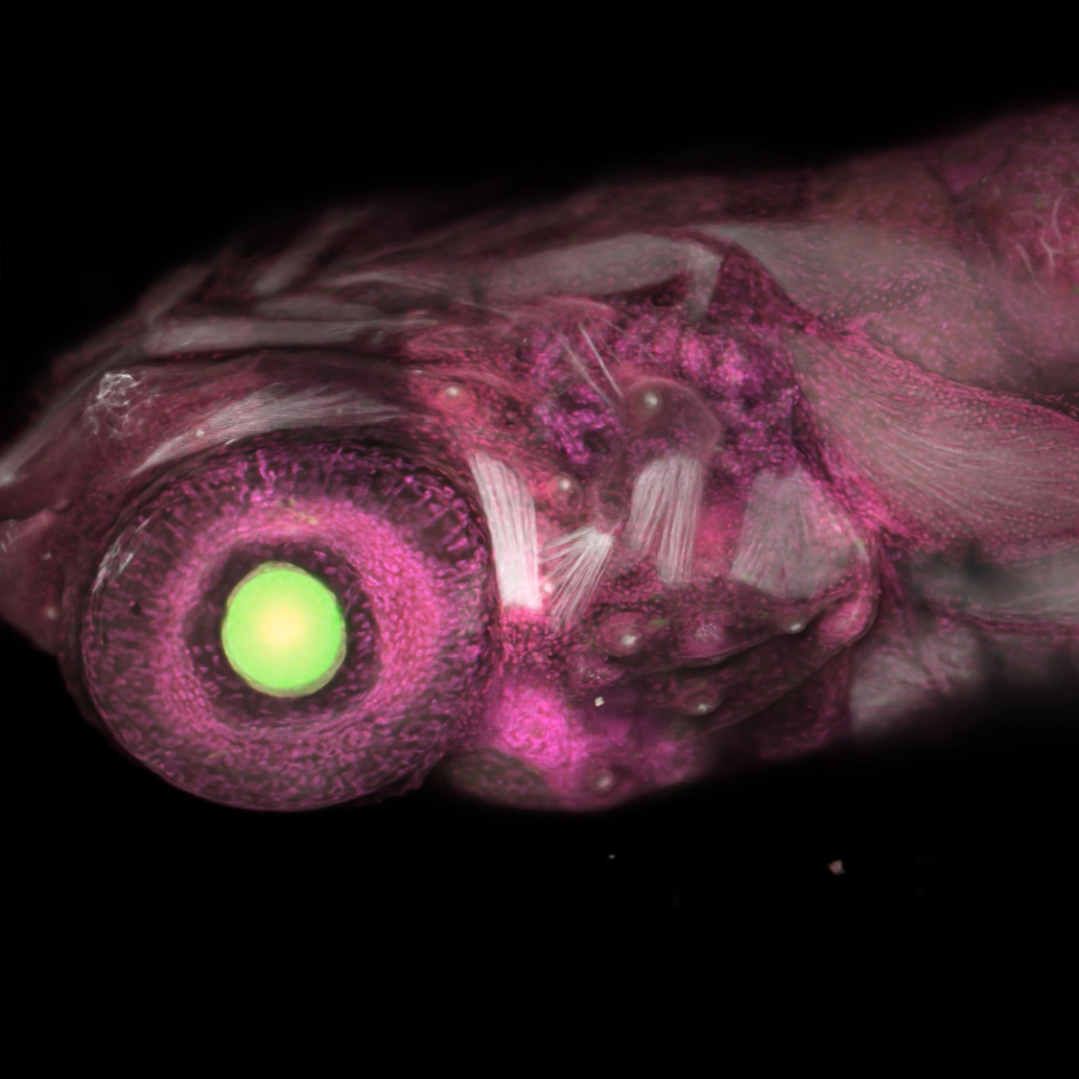
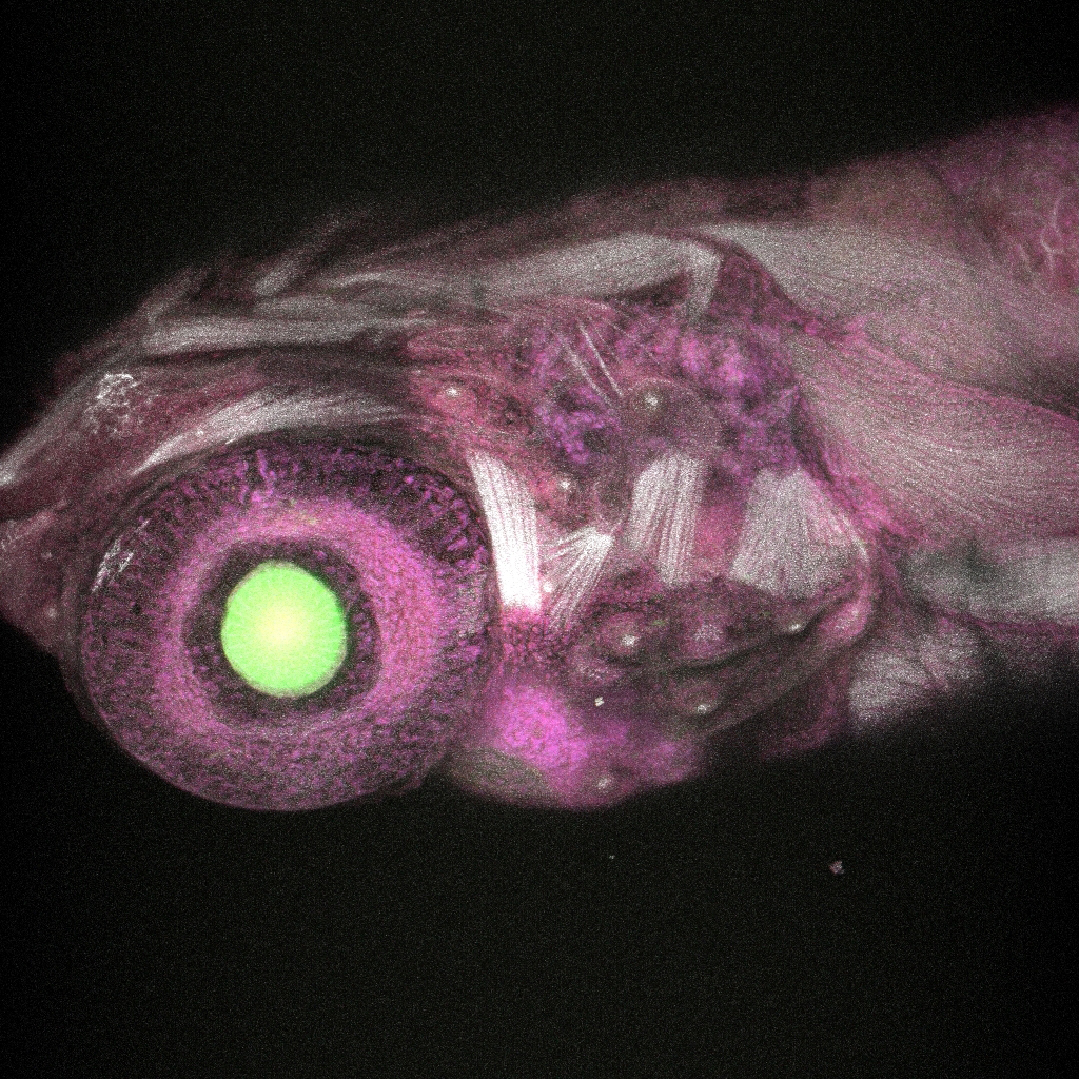
Maximum intensity projection resonant confocal image of multi-labeled Danio sp. prepared by Callen Wallace and Mike Calderon, Center for Biological Imaging, University of Pittsburgh for the Quantitative Fluorescence Microscopy (QFM) Course.
Sources of noise in resonant confocal imaging
Resonant scanning confocal, like all imaging modalities, captures images with signal and also a noise component. Since read noise is effectively negligible by the use of photomultiplier tubes (PMTs), and the dark current noise also minimal due to extremely short exposures (20x shorter than traditional scanning), shot noise is the main contributor of noise to resonant confocal images.
Shot noise is the result of discreetly sampling and digitizing emitting photons from the sample. When light levels are low, the uncertainty due to Poisson distribution of the light flux plays a significant role in the output image.
Denoise.AI works by identifying and removing the shot noise component from resonant confocal images.
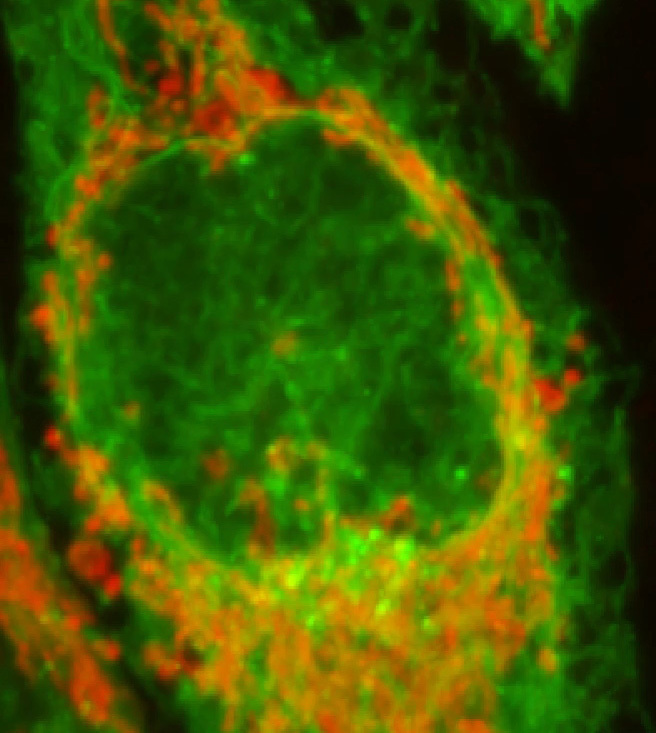
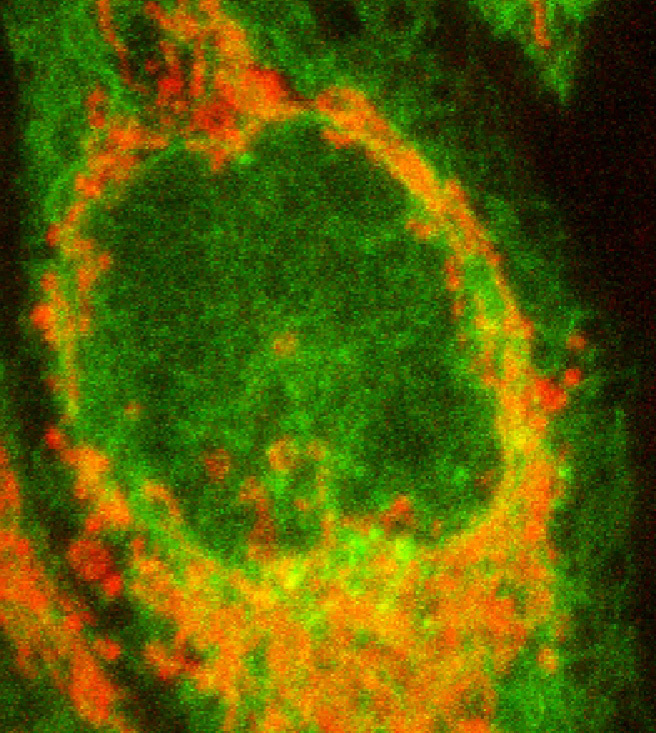
Key Advantages to using Denoise.AI
Resonant scanning works by sinusoidally driving the confocal scanning mirror at very high frequency, resulting in ultra short (tens of nanoseconds) dwell times. These ultra short dwell times are extremely favorable to preventing photo damage and to increasing specimen viability for long term imaging.
Resonant scanning also means samples which move extremely fast can still be acquired with Nyquist sampling frequency for purposes of object tracking.
While resonant scanning at very short exposure times usually requires line averaging to reduce Poisson shot noise contributions, users now instead can employ Denoise.AI to remove the shot noise contribution.
The results are multi-fold:
- Fewer imaging loops results in a longer imaging duration (e.g., not requiring a 4-pass average means one can acquire 4x more images, extending the time-lapse duration or shortening the time-lapse interval).
- Sampling frequency (speed) increases with less averaging, allowing the acquisition of faster biological events.
- Acquisitions with low signal are appreciably improved due to removal of Poisson shot noise.
Drosophila sp. larvae sample provided by Amicia Elliot, PhD, NIH/NIMH, Bethesda MD. Acquired at the Quantitative Fluorescence Microscopy Course 2019.
Caco-2 cells stably expressing H2B-GFP, cultured in Matrigel. Image courtesy of Sally Cheung, Pelletier Lab, Department of Molecular Genetics, University of Toronto.
Quantitative output
Since deep learning is employed to recognize and remove shot noise, the input image data should be of sufficient signal-to-noise ratio (SNR) such that shot noise can be differentiated from the signal. If SNR is sufficient, the variance in intensities due to the Poisson shot noise contribution can be removed.
NIS-Elements provides real time tools to measure signal-to-noise ratio in images to assist in assessing image quality. Importantly however, even images highly corrupted by shot noise can be greatly improved by denoising, allowing users to see structures, track objects over time, or assist in finding and focusing on rapidly moving objects.