Notes d'application
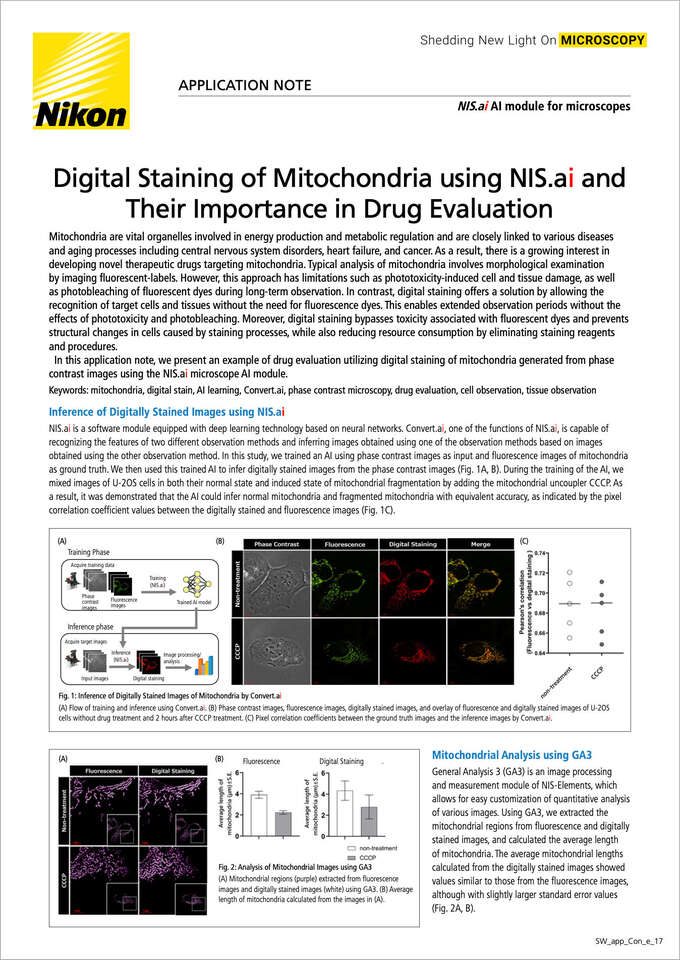
Digital Staining of Mitochondria using NIS.ai and Their Importance in Drug Evaluation
décembre 2023
Mitochondria are vital organelles involved in energy production and metabolic regulation and are closely linked to various diseases and aging processes including central nervous system disorders, heart failure, and cancer. As a result, there is a growing interest in developing novel therapeutic drugs targeting mitochondria. Typical analysis of mitochondria involves morphological examination by imaging fluorescent-labels. However, this approach has limitations such as phototoxicity-induced cell and tissue damage, as well as photobleaching off fluorescent dyes during long-term observation. In contrast, digital staining offers a solution by allowing the recognition of target cells and tissues without the need for fluorescence dyes. This enables extended observation periods without the effects of phototoxicity and photobleaching. Moreover, digital staining bypasses toxicity associated with fluorescent dyes and prevents structural changes in cells caused by staining processes, while also reducing resource consumption by eliminating staining reagents and procedures.
In this application note, we present an example of drug evaluation utilizing digital staining of mitochondria generated from phase contrast images using the NIS.ai microscope AI module.
Inference of Digitally Stained Images using NIS.ai
NIS.ai is a software module equipped with deep learning technology based on neural networks. Convert.ai, one of the functions of NIS.ai, is capable of recognizing features of two different observation methods and inferring images obtained with one observation method based on images obtained with the other observation method. In this study, we trained an AI using phase contrast images as input and fluorescence images of mitochondria as ground truth. We then used this trained AI to infer digitally stained images from the phase contrast images (Fig. 1A, B). During the training of the AI, we mixed images of U-2OS cells in both their normal state and induced state of mitochondrial fragmentation by adding the mitochondrial uncoupler CCCP. As a result, it was demonstrated that the AI could infer normal mitochondria and fragmented mitochondria with equivalent accuracy, as indicated by the pixel correlation coefficient values between the digitally stained and fluorescence images (Fig. 1C).

Fig. 1: Inference of Digitally Stained Images of Mitochondria by Convert.ai
(A) Flow of training and inference using Convert.ai. (B) Phase contrast images, fluorescence images, digitally stained images, and overlay of fluorescence and digitally stained images of U-2OS cells without drug treatment and 2 hours after CCCP treatment. (C) Pixel correlation coefficients between the ground truth images and the inference images by Convert.ai.
Mitochondrial Analysis using GA3
General Analysis 3 (GA3) is an image processing and measurement module of NIS-Elements, which allows for easy customization of quantitative analysis of various images. Using GA3, we extracted the mitochondrial regions from fluorescence and digitally stained images, and calculated the average length of mitochondria. The average mitochondrial lengths calculated from the digitally stained images showed values similar to those from the fluorescence images, although with slightly larger standard error values (Fig. 2A, B).

Fig. 2: Analysis of Mitochondrial Images using GA3
(A) Mitochondrial regions (purple) extracted from fluorescence images and digitally stained images (white) using GA3. (B) Average length of mitochondria calculated from the images in (A).
Application of Digital Staining Technology to Drug Evaluation
Using fluorescence images and digitally stained images, we performed dose-dependent and time-dependent evaluations of the toxicity of oligomycin, an inhibitor of mitochondrial ATP synthesis. Data from 10 fields of view were analyzed for each condition. Similar to fluorescence images, we were able to observe dose-dependent fragmentation of mitochondria following treatment with oligomycin in the digitally stained images (Fig. 3A). Furthermore, quantitative analysis using GA3 revealed significant differences in the average length of mitochondria between different concentrations, similar to the results obtained using fluorescence images (Fig. 3B). Additionally, the digital staining method enabled time-lapse observation of the same sample, which was difficult with fluorescence observation due to phototoxicity-induced mitochondrial fragmentation (Fig. 3C, D).


Fig. 3: Evaluation of Oligomycin Toxicity and Phototoxicity to Mitochondria by Time-lapse Observation
(A) Phase contrast images, mitochondrial staining fluorescence images, and digitally stained images of U-2OS cells treated with oligomycin for 2 hours. (B) Average length of mitochondria calculated from fluorescence images and digitally stained images 2 hours after oligomycin treatment. Data from 10 fields of view were analyzed. (**** p < 0.0001, One-way ANOVA and Tukey's). (C) Percentage change in mitochondrial length calculated from fluorescence images and digitally stained images inferred from phase contrast images, obtained by time-lapse imaging of U-2OS cells without drug treatment. (D) Time-dependent changes in mitochondrial length induced by oligomycin treatment, calculated from digitally stained images. Data from 10 fields of view were analyzed.
Summary
NIS.ai enables the generation of AI models for digital staining analysis without the need for programming knowledge. Generating an AI model with high inference accuracy requires a large amount of image data, which can be acquired by utilizing automated image acquisition routines. The digitally stained images inferred by using NIS.ai can achieve results equivalent to fluorescence images, making them applicable to dose-dependent testing and evaluation of time-dependent changes caused by drug candidates. Therefore, it is expected that these technologies will be widely utilized in the evaluation and research of cells and tissues in various fields such as medicine, life sciences, and environmental sciences.
Experimental condition
Cells
Mitochondria-localized RFP expressing U-2OS cells (Human osteosarcoma)
Observation devices
Microscope: Eclipse Ti2-E
Objective: CFI SR Plan Apochromat IR 60XC WI (NA 1.27)
Acquisition conditions
Calibration: 0.11 µm
Camera settings: Exposure: 70 ms
Binning: 1x1, Scan mode: Fast
Z stack: 0.3 µm x 11 steps
Methods
- After seeding cells in a glass-bottom 24-well plate, culture them overnight at 37℃, 5% CO2.
- After removing the medium, wash twice with medium warmed to 37℃.
- After adding 500 µL of medium containing CCCP, oligomycin and DMSO, place in a stage top incubator.
- Acquire images with Eclipse Ti2-E.
AI training methods
Normal cell images: 239
Drug-treated cell images: 293
Learning times: 2000~3000 times
References
Murphy M et al. “Mitochondria as a therapeutic target for common pathologies”, Nature Reviews Drug Discovery, (2018), 865-886, 17(12)
Jikken Igaku Vol.41 No.5 (special number), 2023
Ounkomol C et al. “Label-free prediction of three-dimensional fluorescence images from transmitted-light microscopy”, Nature Methods, (2018), 917-920, 15(11)
Somani A et al. “Digital Staining of Mitochondria in Label-free Live-cell Microscopy”, Informatik aktuell, (2021), 235-240
Product information
NIS.ai AI module for microscopes
NIS.ai is a module of the NIS-Elements imaging software, which improves the workflow of image processing and analysis using deep learning. Convert.ai, a feature of NIS.ai, can be trained to recognize features of two different observation methods and predict images obtained with one observation method based on images obtained with the other observation method.